Fashion Tech, an innovative and rapidly growing field, has been transforming the way we perceive and experience fashion. This dynamic intersection of fashion and technology has revolutionized the industry, offering exciting possibilities and new ways to engage with consumers. In this article, we will explore the various aspects of Fashion Tech, its impact on retail, sustainability, data analytics, future trends, as well as the challenges and opportunities it presents.
Table of Contents
- I. Introduction
- II. The Role of Technology in Fashion
- III. Fashion Tech in Retail
- IV. Sustainability and Fashion Tech
- V. Fashion Tech and Data Analytics
- VI. Future Trends in Fashion Tech
- VII. Challenges and Opportunities
- VIII. Conclusion
- IX. FAQs
I. Introduction
Fashion Tech refers to the fusion of fashion and technology, where cutting-edge advancements are applied to enhance and revolutionize the traditional fashion industry. It encompasses a wide range of applications, from wearable technology and smart garments to virtual try-ons and sustainable materials. The seamless integration of technology with fashion has not only enhanced the creative process but has also expanded the scope of consumer engagement and retail experiences.
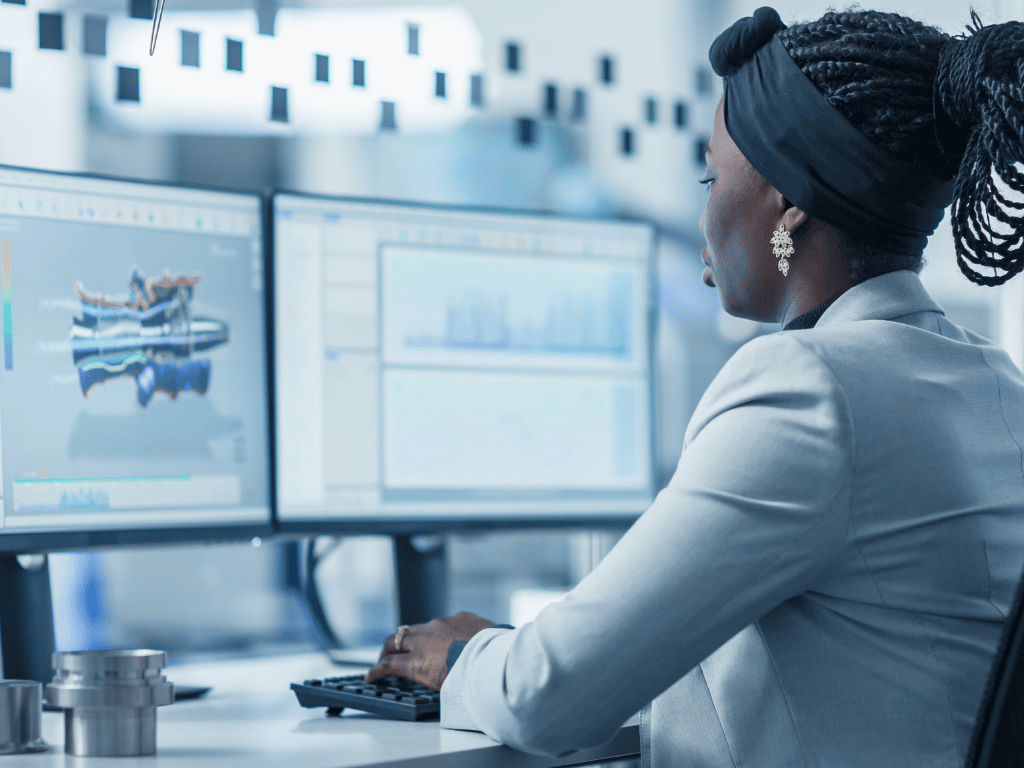
II. The Role of Technology in Fashion
Technology has significantly influenced the world of fashion, introducing new possibilities and pushing the boundaries of creativity. One of the key areas where technology has made a profound impact is in textiles and materials. Innovations such as conductive fabrics, 3D-printed materials, and smart textiles have opened up avenues for designers to create unique and interactive garments.
Digital fashion is another exciting development in the industry. With advancements in augmented reality (AR) and virtual reality (VR), consumers can now try on virtual garments and accessories, allowing them to visualize how an outfit would look and fit without physically trying it on. This technology has not only improved the online shopping experience but has also reduced the rate of returns, benefiting both consumers and retailers.
III. Fashion Tech in Retail
The rise of e-commerce has transformed the way we shop for fashion. Online platforms have provided consumers with a convenient way to explore and purchase a wide range of products from the comfort of their homes. Moreover, the integration of technology has enabled retailers to offer personalized recommendations based on individual preferences and browsing behavior, enhancing the overall shopping experience.
Augmented reality and virtual reality have also found their place in the retail sector. Retailers are leveraging these technologies to create immersive virtual showrooms, allowing customers to virtually try on clothes, experiment with different styles, and even visualize how furniture or accessories would look in their homes. These interactive experiences not only captivate shoppers but also drive engagement and increase conversion rates.
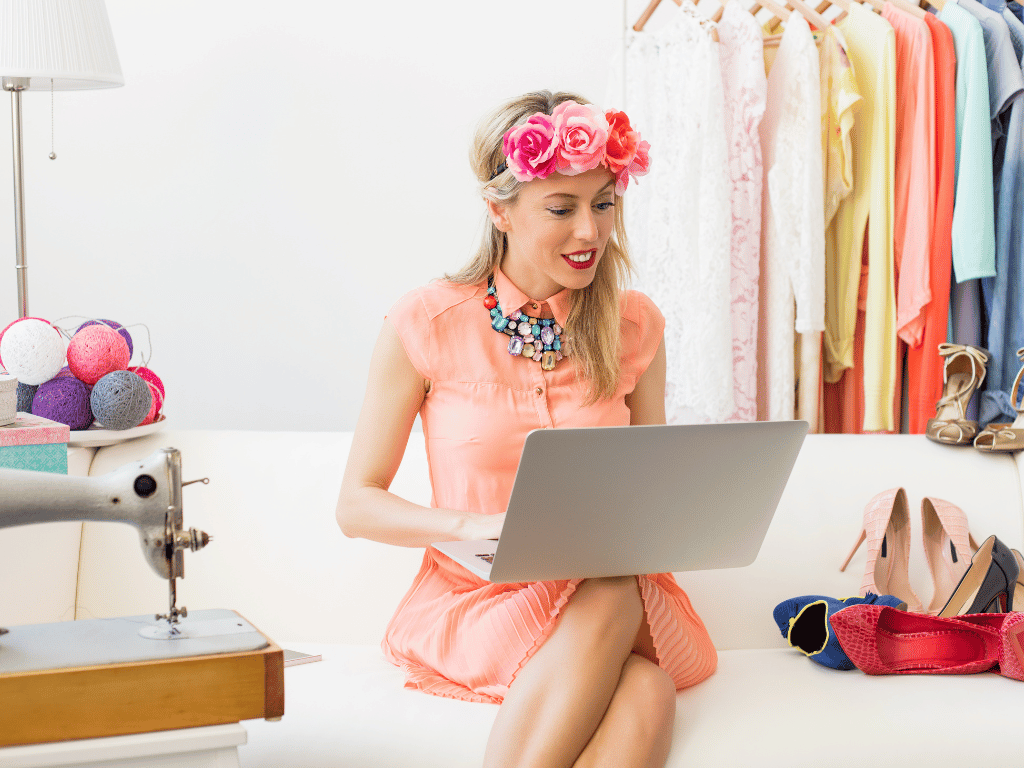
IV. Sustainability and Fashion Tech
In recent years, sustainability has become a pressing concern in the fashion industry. Fashion Tech has played a crucial role in addressing these concerns by offering sustainable alternatives in materials and production processes. Innovations like biofabrication, which involves growing fabrics using cells instead of traditional manufacturing methods, have the potential to significantly reduce the environmental impact of fashion.
Additionally, technologies for tracking and reducing the carbon footprint of garments have emerged. RFID (Radio Frequency Identification) tags and blockchain technology can provide transparency in the supply chain, allowing consumers to trace the origins of their clothes and ensuring ethical and sustainable practices.
Recycling and upcycling technologies have also gained traction in the fashion industry. Advanced recycling processes can transform textile waste into new materials, reducing the reliance on virgin resources. Upcycling, on the other hand, involves repurposing old garments into new, unique pieces, adding value and reducing waste.
V. Fashion Tech and Data Analytics
Data analytics has become an invaluable tool in the fashion industry, enabling brands to gain insights into consumer behavior and market trends. By analyzing vast amounts of data, fashion companies can make informed decisions regarding inventory management, demand forecasting, and personalized marketing strategies.
Customer insights derived from data analytics help designers understand consumer preferences, allowing them to create collections that resonate with their target audience. Trend forecasting models powered by artificial intelligence and machine learning algorithms provide accurate predictions, enabling brands to stay ahead of the curve and meet consumer demands effectively.
Data analytics also plays a crucial role in optimizing the fashion supply chain. By analyzing data related to production, transportation, and inventory, companies can identify bottlenecks, streamline processes, and reduce waste, ultimately improving efficiency and profitability.
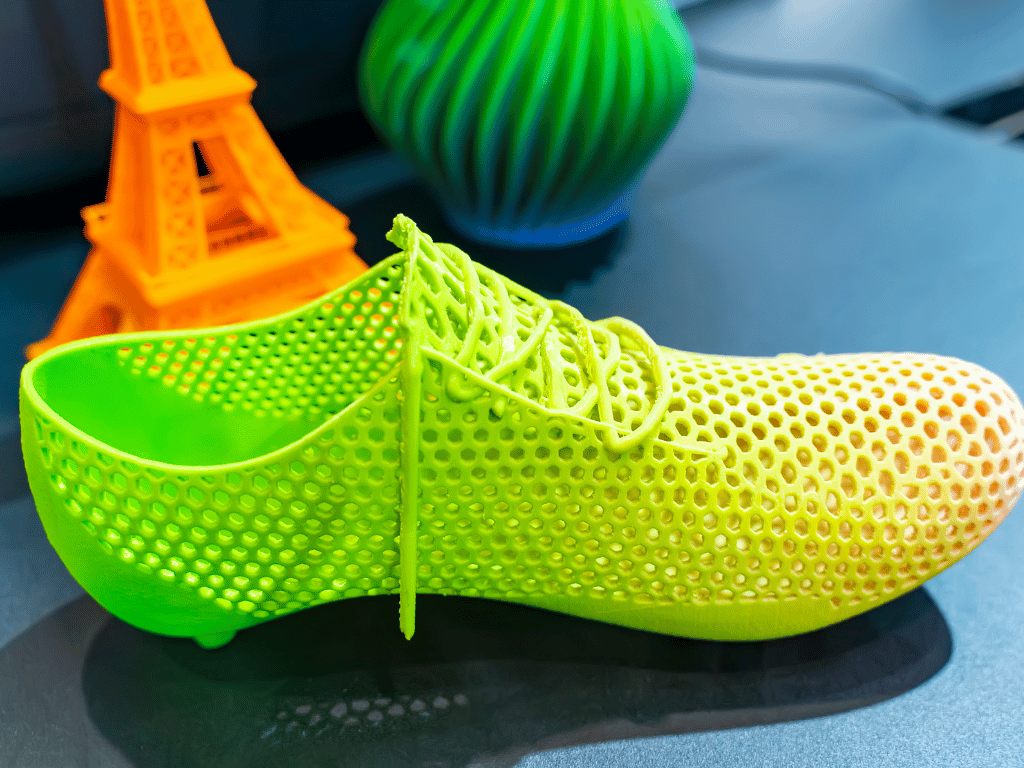
VI. Future Trends in Fashion Tech
The future of Fashion Tech is filled with exciting possibilities. One emerging trend is the use of 3D printing in fashion. This technology allows designers to create intricate and customizable garments, pushing the boundaries of traditional manufacturing. With 3D printing, consumers can have unique, perfectly fitting clothes tailored to their individual measurements.
Artificial intelligence and machine learning will continue to play a significant role in fashion. AI-powered chatbots and virtual stylists can provide personalized recommendations and styling advice to consumers. Machine learning algorithms can analyze fashion trends and consumer preferences, helping brands create targeted marketing campaigns and design products that align with the latest fashion movements.
The Internet of Things (IoT) is another technology poised to transform the fashion industry. Smart garments embedded with sensors can collect data on body temperature, movement, and biometrics, providing valuable insights for fitness, health, and performance optimization. IoT devices can also enable seamless integration between garments and other smart devices, creating a connected and personalized fashion experience.
VII. Challenges and Opportunities
The integration of fashion and technology brings both challenges and opportunities. One of the main challenges is achieving a seamless fusion of fashion aesthetics and technological functionality. Designers need to strike a balance between innovation and wearability, ensuring that technology enhances the fashion experience rather than overshadowing it.
Ethical considerations are also crucial in the realm of Fashion Tech. Ensuring fair and sustainable practices throughout the supply chain, protecting workers’ rights, and addressing privacy concerns are essential for the industry’s long-term success.
Collaborations and partnerships between fashion brands and technology companies present significant opportunities. By combining their expertise, these collaborations can drive innovation, create unique experiences, and shape the future of fashion.
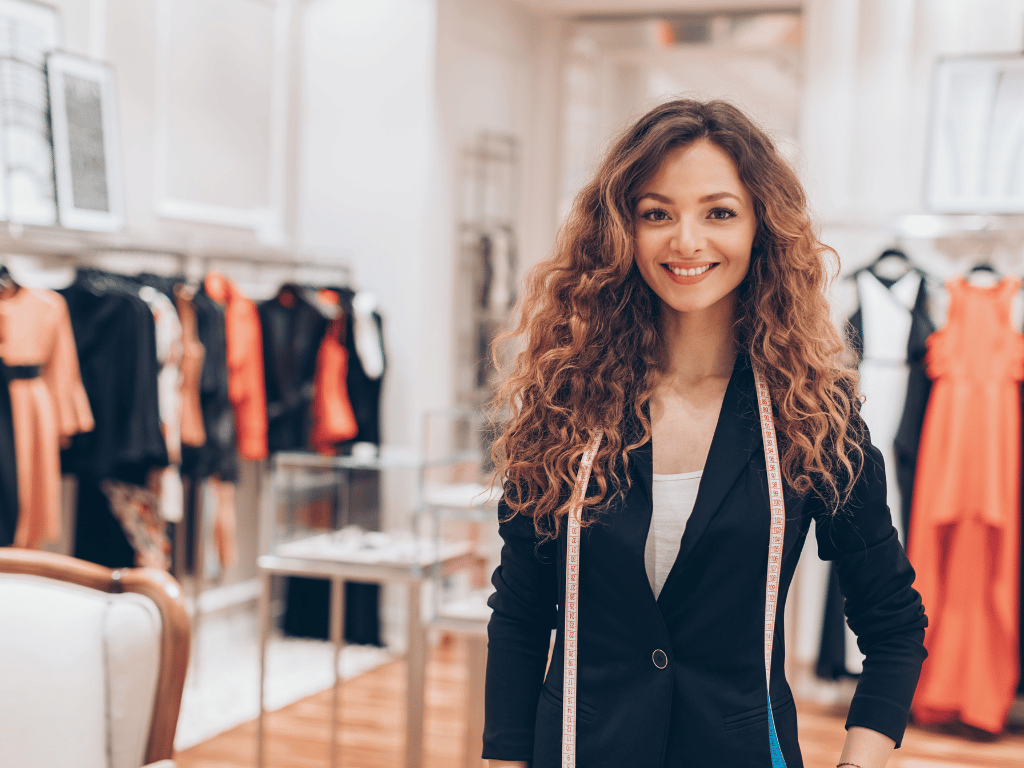
VIII. Conclusion
Fashion Tech is revolutionizing the fashion industry, offering new possibilities, enhancing sustainability, and transforming the way we shop and engage with fashion. Advancements in technology have facilitated personalized experiences, improved supply chain efficiency, and fostered creative innovation. As Fashion Tech continues to evolve, it is crucial for industry stakeholders to embrace the opportunities it presents while addressing challenges such as maintaining a balance between fashion aesthetics and technology, ensuring ethical practices, and fostering collaborations.
Fashion Tech has the potential to shape the future of fashion by providing unique and immersive experiences, sustainable solutions, and data-driven insights. It empowers both designers and consumers, opening up a world of endless creativity and personalized fashion choices. As we move forward, the collaboration between fashion and technology will continue to reshape the industry and redefine the way we experience and interact with fashion.
IX. FAQs
1. How does Fashion Tech impact sustainability in the fashion industry? Fashion Tech offers sustainable alternatives in materials and production processes, such as biofabrication and recycling technologies. It also enables the tracking and reduction of environmental impact through RFID tags and blockchain technology.
2. How does data analytics contribute to the fashion industry? Data analytics provides valuable insights into consumer behavior, trend forecasting, and supply chain optimization. It helps designers create collections that align with consumer preferences and enables brands to make informed decisions regarding inventory management and personalized marketing strategies.
3. What are some future trends in Fashion Tech? Future trends in Fashion Tech include 3D printing for customizable garments, artificial intelligence for personalized recommendations, and the Internet of Things for smart garments and connected fashion experiences.
4. What are the challenges of integrating fashion and technology? One of the main challenges is achieving a balance between fashion aesthetics and technological functionality. Ethical considerations and privacy concerns also need to be addressed to ensure fair and sustainable practices in the industry.
5. How can collaborations and partnerships drive innovation in Fashion Tech? Collaborations between fashion brands and technology companies bring together expertise from both fields, fostering innovation, creating unique experiences, and shaping the future of fashion.
Read more about Fashion Tech: Innovations and Impacts 2023
Thank you for reading this comprehensive article on Fashion Tech. We hope you found it informative and inspiring. Stay tuned for the latest advancements in Fashion Tech and explore the exciting possibilities that lie at the intersection of fashion and technology.
Read News and other featured articles on www.yessiey.com